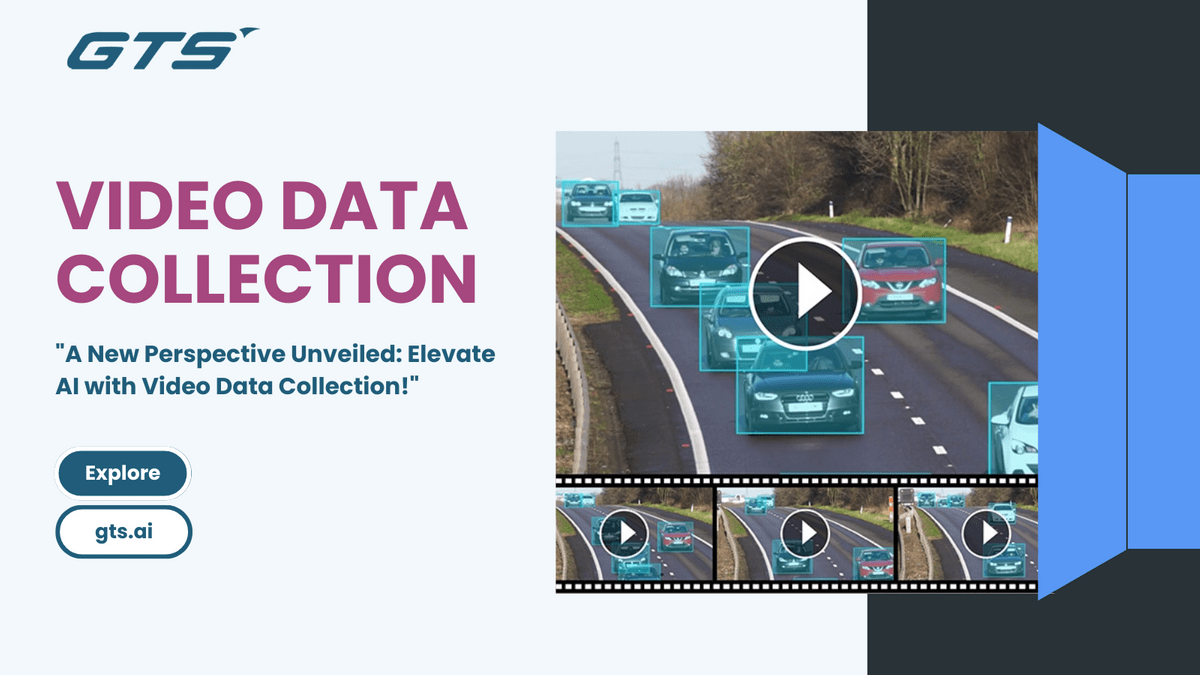
Introduction:
In the ever-evolving field of deep learning, video data has become an invaluable source of insights and information. From surveillance and security applications to entertainment and sports analytics, the potential of video data is vast and promising. However, to harness the power of video data, businesses must leverage robust deep learning frameworks like TensorFlow. In this blog, we explore the significance of Video Data Collection and how TensorFlow enables companies to perform cutting-edge deep learning on video data, driving innovation and unlocking the full potential of visual intelligence.
The Power of Video Data Collection:
Video data provides a wealth of information that goes beyond what can be captured in still images or text. The ability to observe motion, temporal patterns, and dynamic interactions makes video data a treasure trove for AI and deep learning applications. Collecting video data from diverse sources and scenarios allows businesses to gain valuable insights, identify anomalies, and make informed decisions based on visual information.
Understanding TensorFlow for Deep Learning:
TensorFlow is an open-source deep learning framework developed by Google Brain. Known for its flexibility, scalability, and user-friendly interface, TensorFlow has become a go-to choice for building and training complex neural network models. It provides a range of tools and functionalities specifically tailored for working with video data, making it an ideal choice for deep learning projects in this domain.
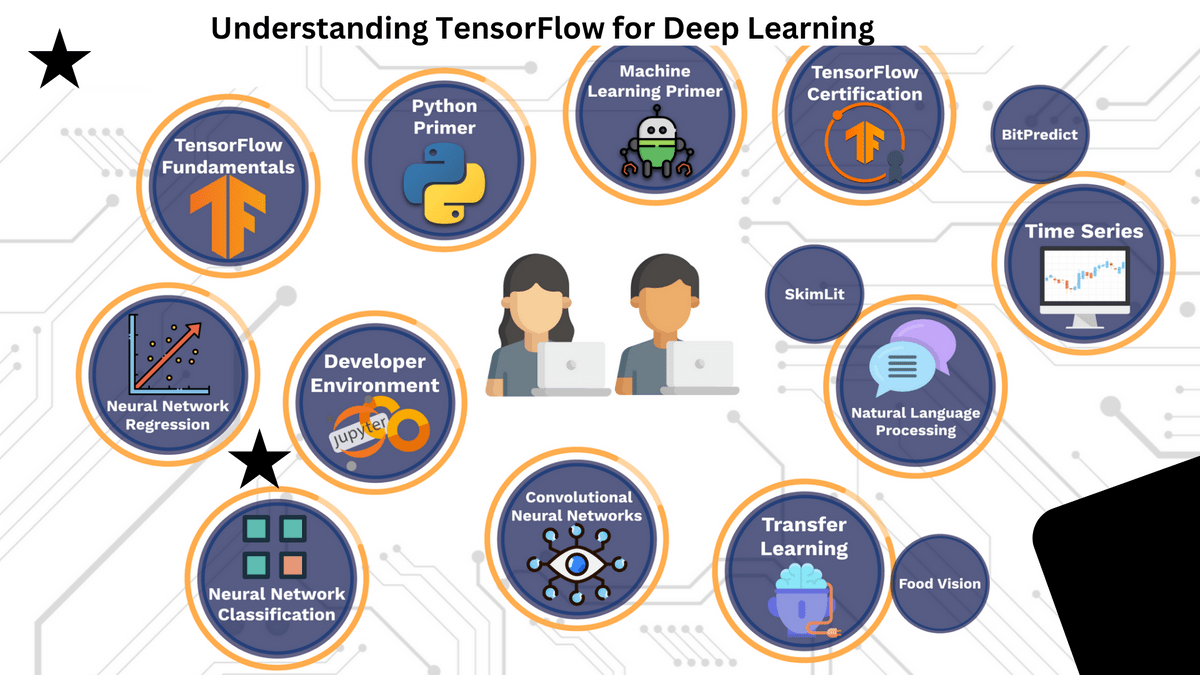
Leveraging TensorFlow for Video Data:
- Video Data Preprocessing: TensorFlow offers a variety of tools for preprocessing video data. This includes techniques for frame extraction, resizing, and normalisation to ensure that the data is in a suitable format for training deep learning models.
- Video Classification: TensorFlow enables businesses to build video classification models capable of categorising videos into various classes or labels. Applications range from activity recognition to sentiment analysis in video content.
- Object Detection in Video: Using TensorFlow's object detection APIs, businesses can detect and track objects within video frames. This has applications in surveillance, autonomous vehicles, and video analytics.
- Video Captioning: TensorFlow facilitates video captioning, where deep learning models can generate Text Data Collection descriptions for video content, enhancing accessibility and understanding.
- Action Recognition: TensorFlow can be used to build action recognition models that identify and classify human actions and gestures in video data.
- Anomaly Detection: TensorFlow's anomaly detection capabilities can be applied to video data to identify irregular patterns and events, useful in areas like security and fraud detection.
- Temporal Models: TensorFlow supports the creation of temporal models, enabling the processing of time-series video data, such as continuous video streams or videos with a sequence of actions.
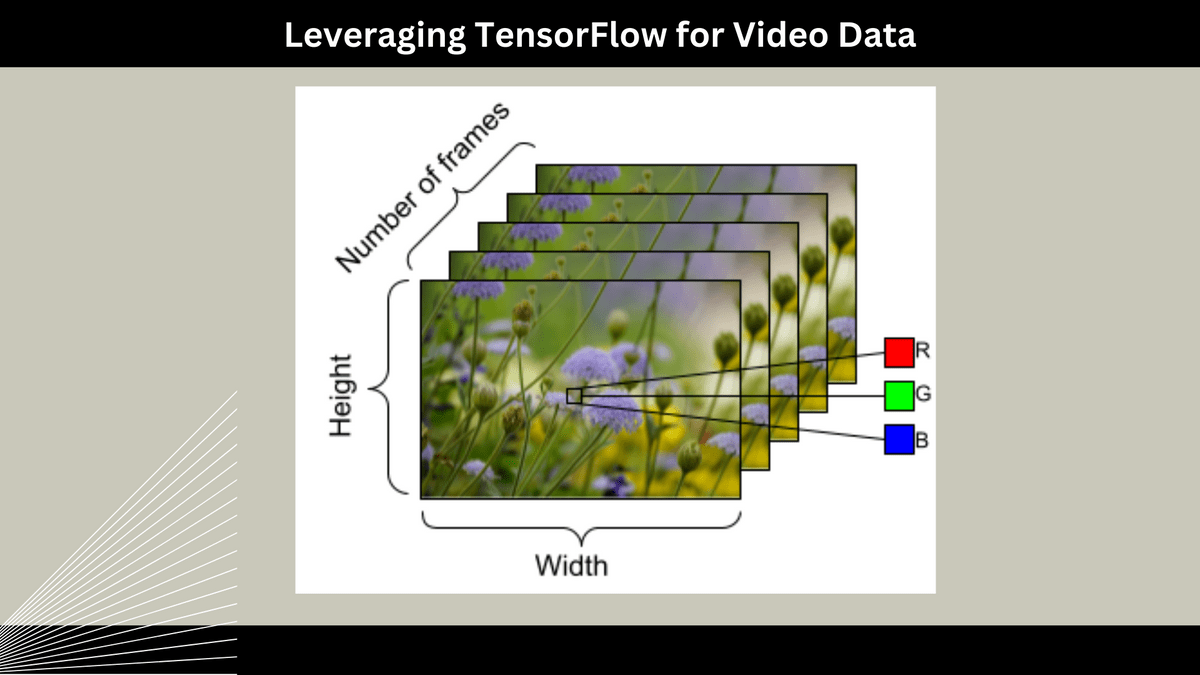
Conclusion:
The value of video data collection in today's data-driven world cannot be overstated. The ability to leverage deep learning on video data opens up a realm of possibilities, from enhancing security and surveillance to gaining actionable insights in various industries. TensorFlow stands as a powerful toolset that allows businesses to perform cutting-edge deep learning on video data. By leveraging TensorFlow's capabilities for video data preprocessing, classification, object detection, captioning, and more, businesses can unlock the full potential of visual intelligence, driving innovation and staying ahead in the fast-paced world of AI and deep learning.
GTS.AI And Video Data Collection
Globose Technology Solutions expertise and experience in video data collection. Consider their track record, client testimonials, or case studies to understand their capabilities and successful projects in this domain.GTS.AI, explore their website, review their portfolio or case studies, and potentially engage in direct communication to discuss your specific video data collection requirements and evaluate their suitability