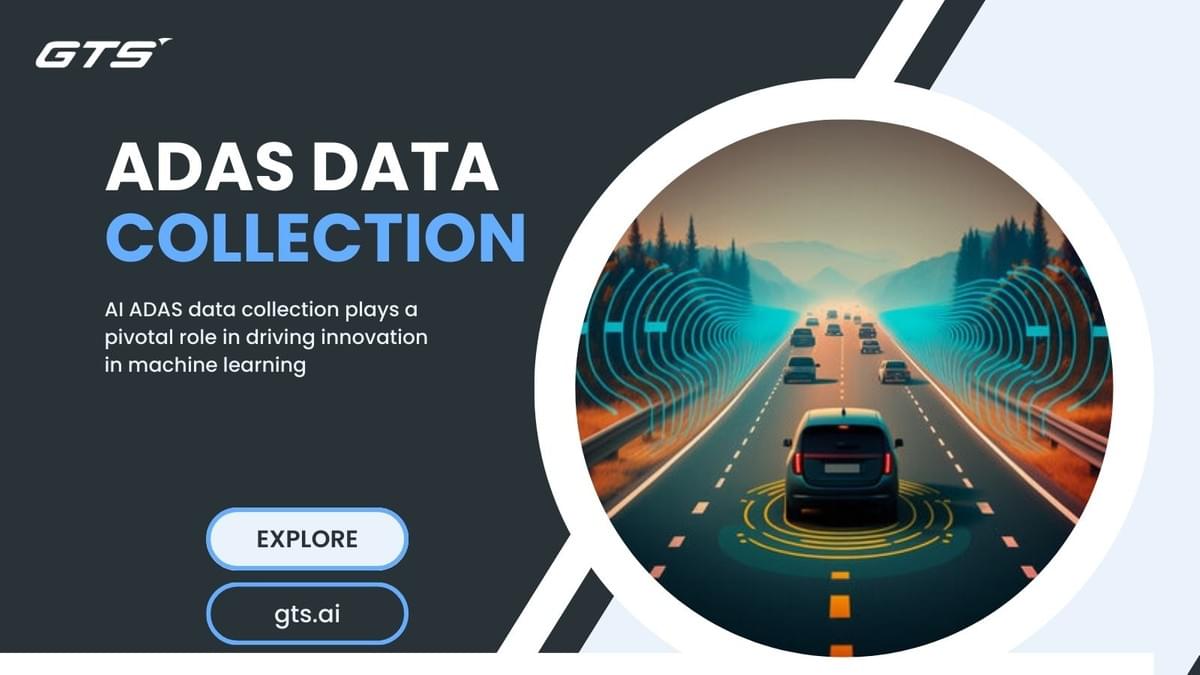
Introduction:
The journey towards autonomous driving is powered by the fusion of cutting-edge technologies, and at the heart of this transformation lies Advanced Driver Assistance Systems (ADAS). These systems, built on the principles of artificial intelligence and machine learning, play a pivotal role in enabling vehicles to perceive their surroundings and make informed decisions. The cornerstone of ADAS development is high-quality data, which serves as the eyes and ears for teaching machines to drive. In this blog, we delve into the significance of ADAS Data Collection and the power of observation in shaping the future of automotive technology.
ADAS: Bridging the Gap between Human and Machine Driving
Advanced Driver Assistance Systems have revolutionised the automotive industry by introducing a new paradigm of safety and convenience. ADAS technologies, such as lane departure warnings, adaptive cruise control, and automatic emergency braking, assist drivers in various aspects of their journey. These systems rely on a vast array of sensors, including cameras, LiDAR, radar, and ultrasonic sensors, to gather data from the vehicle's surroundings.
The Crucial Role of Data in ADAS Development:
For ADAS to function effectively, it requires access to a diverse and comprehensive dataset, containing real-world driving scenarios. This data is instrumental in training machine learning models that power the decision-making capabilities of ADAS systems. The data collection process involves capturing various driving scenarios, including urban streets, highways, adverse weather conditions, and complex traffic situations.
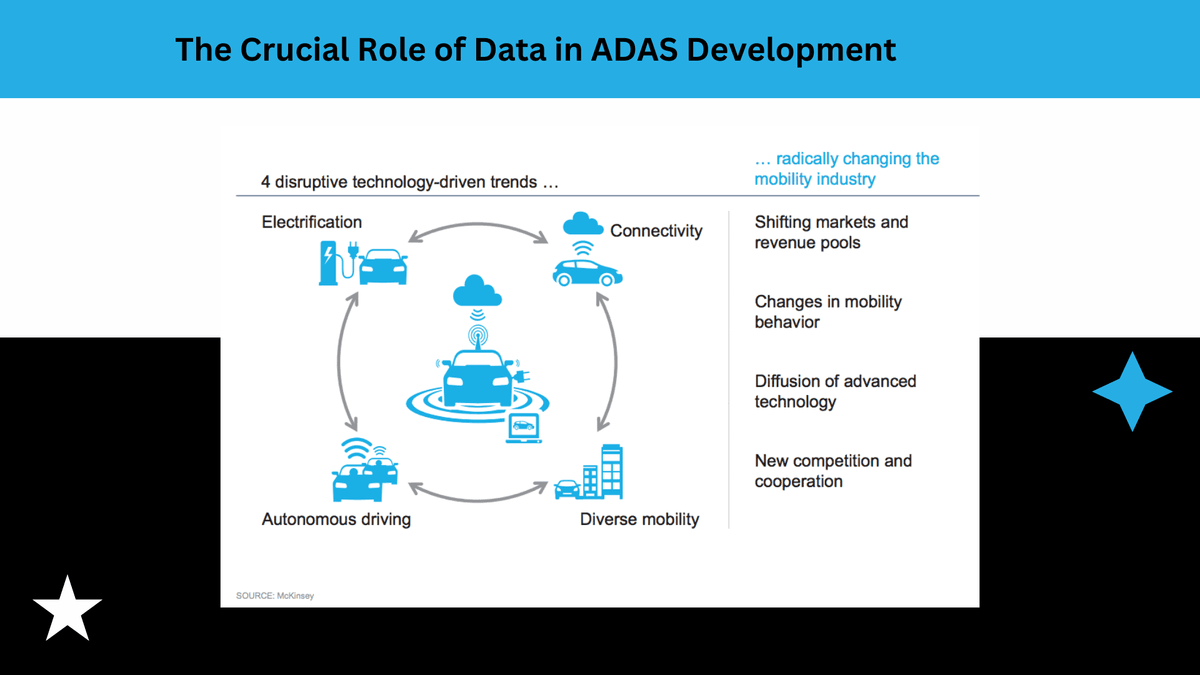
Real-World Data for Realistic Machine Learning:
The power of observation lies in the use of real-world data to provide machines with a diverse and representative view of driving scenarios. This data-driven approach ensures that ADAS systems are equipped to handle a wide range of situations, making them more robust and reliable on the road. From pedestrians crossing the street to unexpected roadblocks, the data collected plays a crucial role in simulating the complexities of real driving.
Annotated Data: Enabling Supervised Learning
To teach machines to drive, human expertise comes into play through the process of data annotation. Skilled annotators meticulously label the collected data, marking objects, lane boundaries, traffic signs, and other critical elements. This ADAS Annotation serves as the ground truth for supervised learning algorithms, allowing machines to learn from human-provided examples and make informed decisions in similar situations.
Ethical Considerations in Data Collection:
While ADAS data collection holds immense potential, it also comes with ethical responsibilities. Ensuring data privacy and security is paramount, and companies involved in ADAS data collection must comply with regulations and industry standards. Anonymizing personal information and safeguarding sensitive data are essential steps to maintain public trust in these transformative technologies.
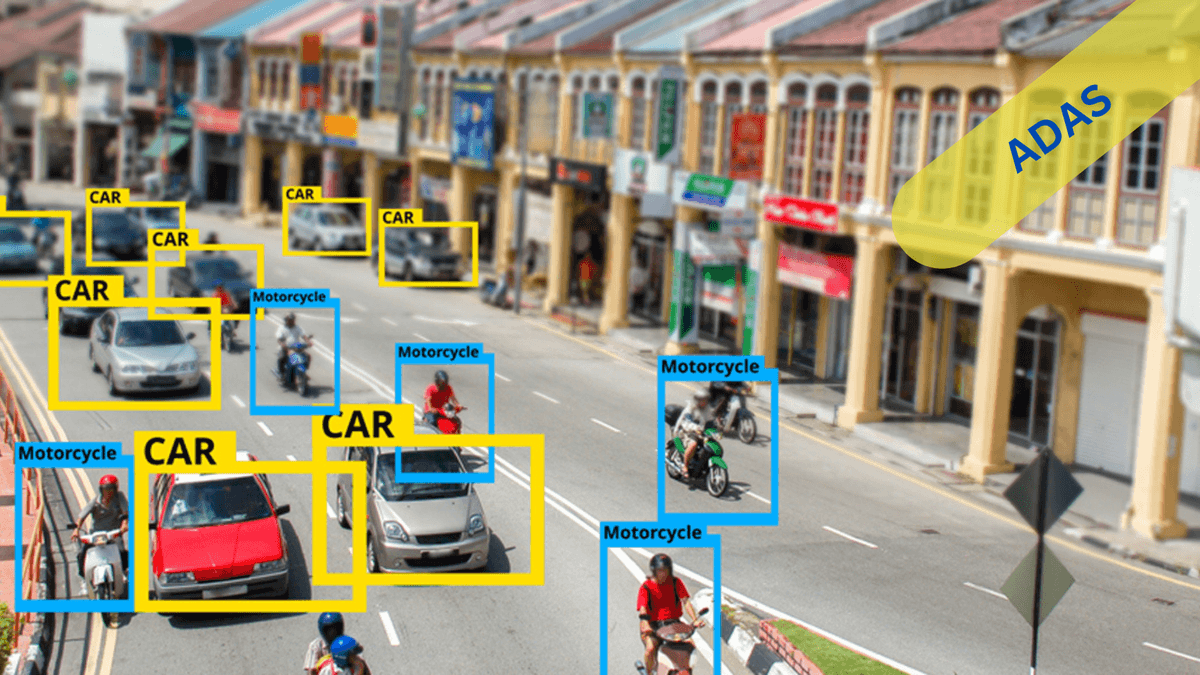
Continuous Learning and Improvement:
The power of observation doesn't end with data collection and model training. ADAS systems require continuous learning and improvement to adapt to changing road conditions and driving patterns. Real-time data streams from connected vehicles and fleet monitoring help update and enhance the performance of ADAS algorithms, making them even more effective over time.
Conclusion:
ADAS data collection is at the heart of teaching machines to drive and the pursuit of autonomous driving. The power of observation, manifested in high-quality, real-world data, forms the bedrock for building robust and reliable ADAS systems. As the automotive industry advances towards a safer and more efficient future, companies specialising in ADAS data collection play a crucial role in shaping the evolution of autonomous driving. Through ethical data practices, continuous learning, and cutting-edge technology, we stand on the cusp of a transformative era, where machines and humans work in harmony to redefine the concept of mobility.
ADAS Data Collection and GTS.AI
Globose Technology Solutions should focus on collecting data from a wide range of driving scenarios and environments to ensure comprehensive coverage. This includes various road types, weather conditions, and traffic situations.GTS.AI should have robust processes for annotating and labeling the collected data. Accurate and detailed annotations are crucial for training ML models effectively.