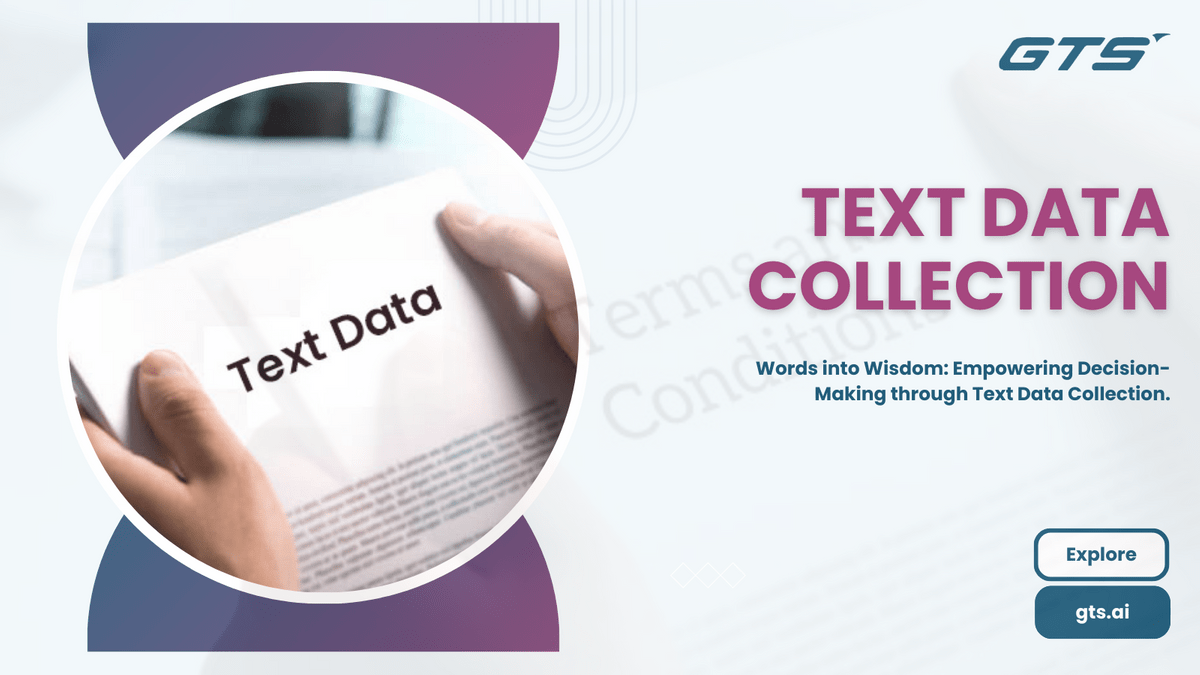
Introduction:
In the realm of machine learning (ML), the old adage "garbage in, garbage out" holds true. The quality and relevance of data play a critical role in the performance of ML models. When it comes to text-based applications, such as natural language processing (NLP), accurate and diverse text data collection is essential. Globose Technology Solutions Pvt Ltd (GTS) understands the nuances of Text Data Collection and its impact on ML success. In this blog post, we delve into the strategies for effective text data collection, showcasing how GTS is at the forefront of curating high-quality text datasets that drive NLP innovations.
The Challenge of Text Data:
Textual data is vast, varied, and unstructured. It spans languages, dialects, styles, and domains, making the collection process complex. For ML models to derive insights and make predictions, the data must be cleaned, organized, and annotated, highlighting the significance of data collection strategies.
GTS's Expertise in Text Data Collection:
GTS excels in the art of text data collection, recognizing that a comprehensive dataset forms the foundation of successful ML applications. Their approach is a blend of technology and human curation. By utilizing advanced web crawling techniques, text extraction tools, and a team of linguistic experts, GTS ensures that the collected text data is accurate, diverse, and reflective of real-world language patterns.

Strategies for Effective Text Data Collection:
- Diverse Sources: GTS understands that ML models thrive on diversity. They collect text data from various sources, including websites, social media, news articles, and domain-specific documents. This diverse pool of data ensures that ML models can handle a wide array of language variations and topics.
- Data Validation: Text data collection involves validation to ensure quality and accuracy. GTS employs linguistic experts to validate and annotate the collected data, correcting errors and adding context. This meticulous process enhances the value of the dataset.
- Annotation and Labeling: Some NLP Text to Speech Dataset tasks require labeled data, such as sentiment analysis or intent recognition. GTS's annotation process involves adding labels to text data, enabling ML models to learn patterns and make predictions.
- Privacy and Ethics: In an age of data privacy concerns, GTS prioritizes ethical data collection practices. They ensure that collected data respects user privacy, complies with regulations, and maintains data security.
Driving NLP Innovations:
GTS's dedication to high-quality text data collection fuels innovation across industries:
- Customer Support: NLP-powered chatbots and virtual assistants enhance customer support experiences by understanding and responding to user queries.
- Sentiment Analysis: ML models trained on diverse text data can accurately determine the sentiment behind user reviews, social media posts, and customer feedback.
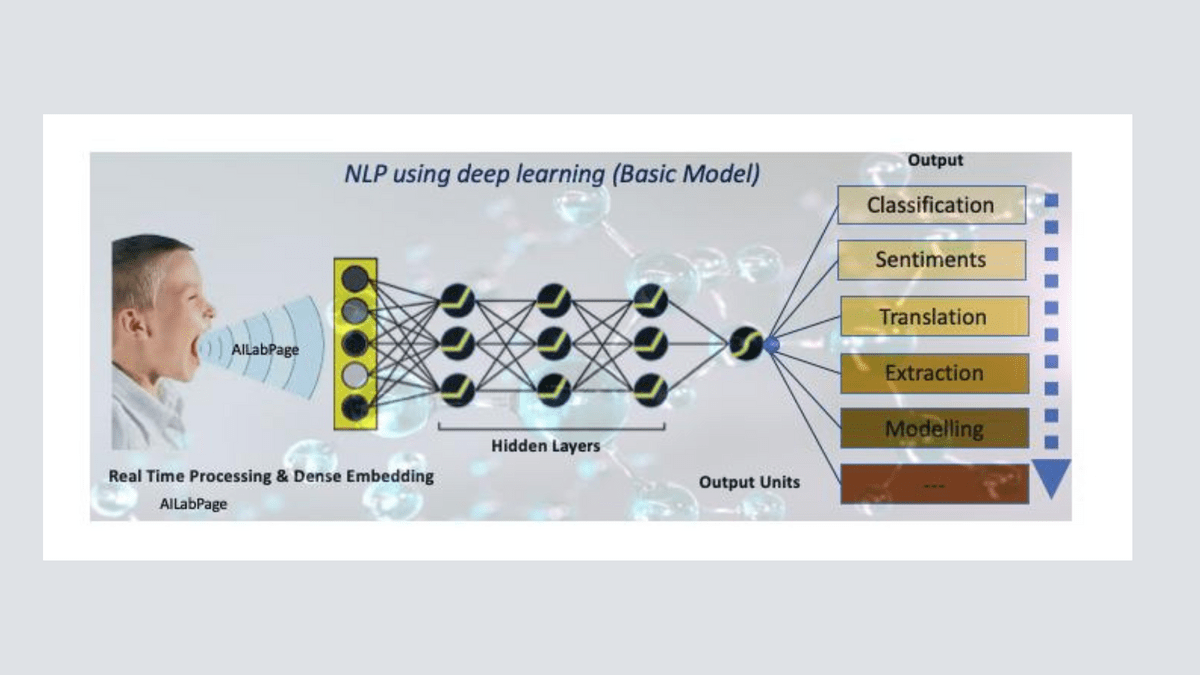
Conclusion:
Text data collection is an art that shapes the NLP landscape. Globose Technology Solutions Pvt Ltd (GTS) is a pioneer in curating high-quality text datasets that drive ML innovation. Through a blend of technology, linguistic expertise, and ethical considerations, GTS creates datasets that lay the groundwork for NLP applications that understand, interpret, and communicate in human-like ways. As ML continues to redefine our interaction with text, GTS remains at the forefront, weaving the threads of language into the fabric of cutting-edge technology.