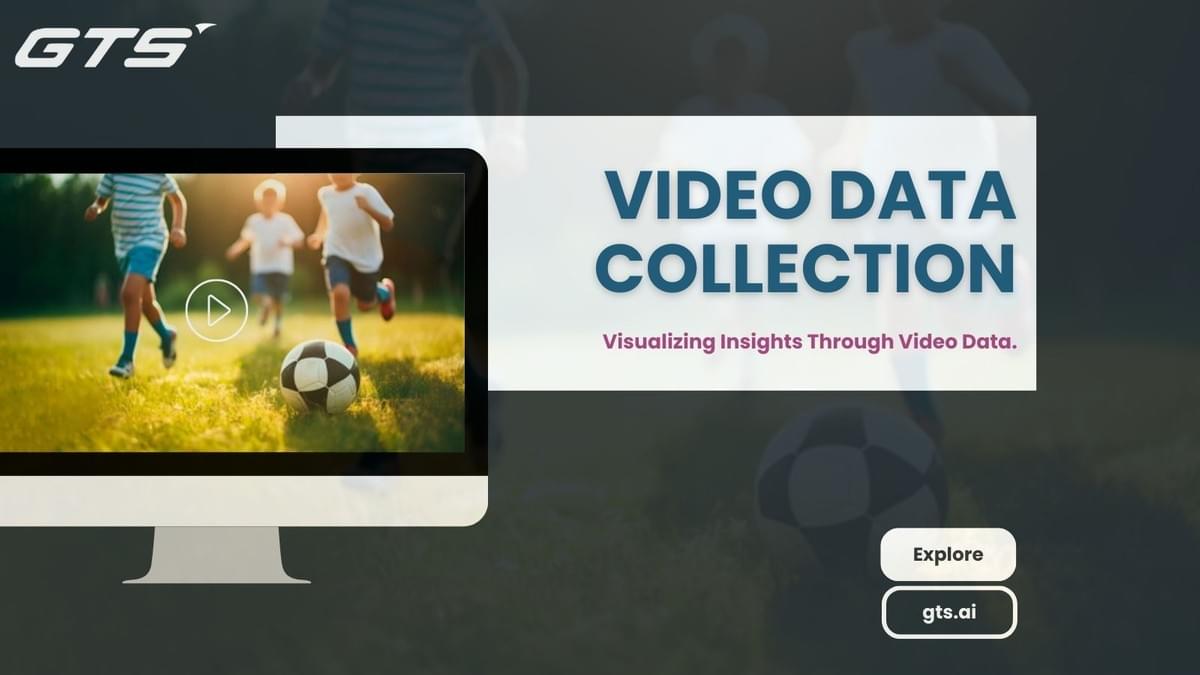
Introduction:
In the exciting world of Machine Learning (ML), the fusion of multiple data modalities has opened up new avenues for advanced AI applications. Multimodal Learning, a cutting-edge approach, involves combining information from various sources such as video, audio, and text to enhance the capabilities of ML models. Video Data Collection, in particular, plays a pivotal role in multimodal learning, providing rich visual information that complements and enriches audio and textual data. In this blog, we explore the power of multimodal learning and the significance of video data collection in driving AI to new heights.
Understanding Multimodal Learning:
In traditional Machine Learning, models are often trained on single-modal data, such as text or images, to perform specific tasks. Multimodal Learning takes a more holistic approach, integrating information from multiple sources to enhance the understanding and performance of ML models. By combining video, audio, and Text Data Collection, multimodal learning unlocks a wealth of insights and enables AI systems to process information more like humans do.
The Role of Video Data in Multimodal Learning:
Video data is a rich source of visual information, capturing dynamic scenes and activities. When combined with audio and text data, video data enriches the learning process, allowing ML models to extract more comprehensive and contextual insights.
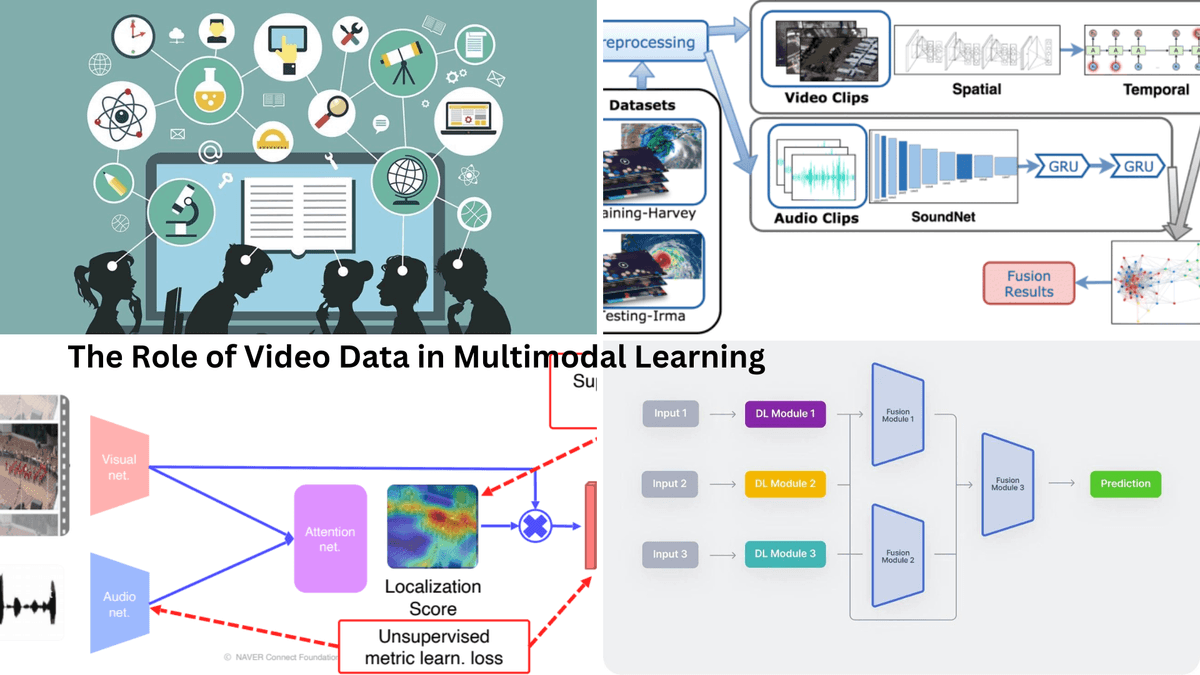
Significance of Video Data Collection:
Visual Contextual Understanding: Video data collection enables ML models to understand visual context and relationships better. For example, combining video with audio can help in recognizing and associating speech with corresponding actions or events.
- Action Recognition: Video data allows ML models to recognize human actions and gestures, a crucial capability for applications such as gesture-based user interfaces or activity recognition in surveillance systems.
- Visual-Audio Synchronisation: Video data collection facilitates synchronising audio and visual data, making it easier for models to align and relate auditory and visual information.
- Visual Sentiment Analysis: Combining video with text data can aid in sentiment analysis, enabling models to understand emotions conveyed through facial expressions and contextual text.
Driving AI Advancements with Multimodal Learning:
- Enhanced Natural Language Processing (NLP): By incorporating video and audio data into NLP tasks, models can gain a deeper understanding of language in real-world contexts, leading to more accurate language processing and comprehension.
- Advanced Virtual Assistants: Multimodal Learning can create more interactive and contextually-aware virtual assistants that can understand and respond to users' commands and queries more intelligently.
- Augmented Reality (AR) and Virtual Reality (VR): Video data, when combined with audio and text, enhances AR and VR experiences, enabling more immersive and realistic simulations.
- Autonomous Systems: Multimodal Learning equips autonomous systems, such as self-driving cars or robots, with a comprehensive understanding of their surroundings, making them safer and more efficient.
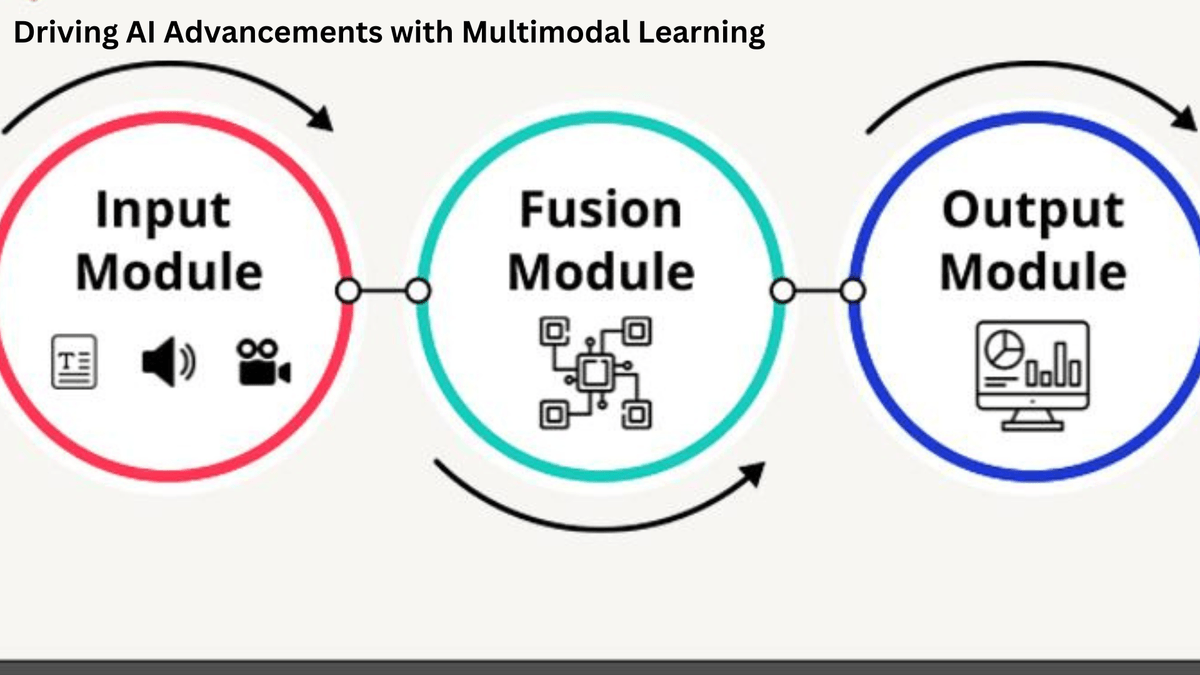
Conclusion:
Multimodal Learning is revolutionising the field of AI, empowering models to process information from various sources, including video, audio, and text, for more sophisticated and contextually-aware outcomes. Video data collection plays a pivotal role in multimodal learning, enriching AI applications with a deeper understanding of the visual world.
How GTS.AI Can Help You?
At Globose Technology Solutions Pvt Ltd (GTS), we understand the importance of high-quality video data collection and offer comprehensive solutions to fuel the success of your multimodal learning projects. By leveraging the power of video data and integrating it with audio and text, you can unlock the full potential of AI and pave the way for innovative and impactful AI applications.