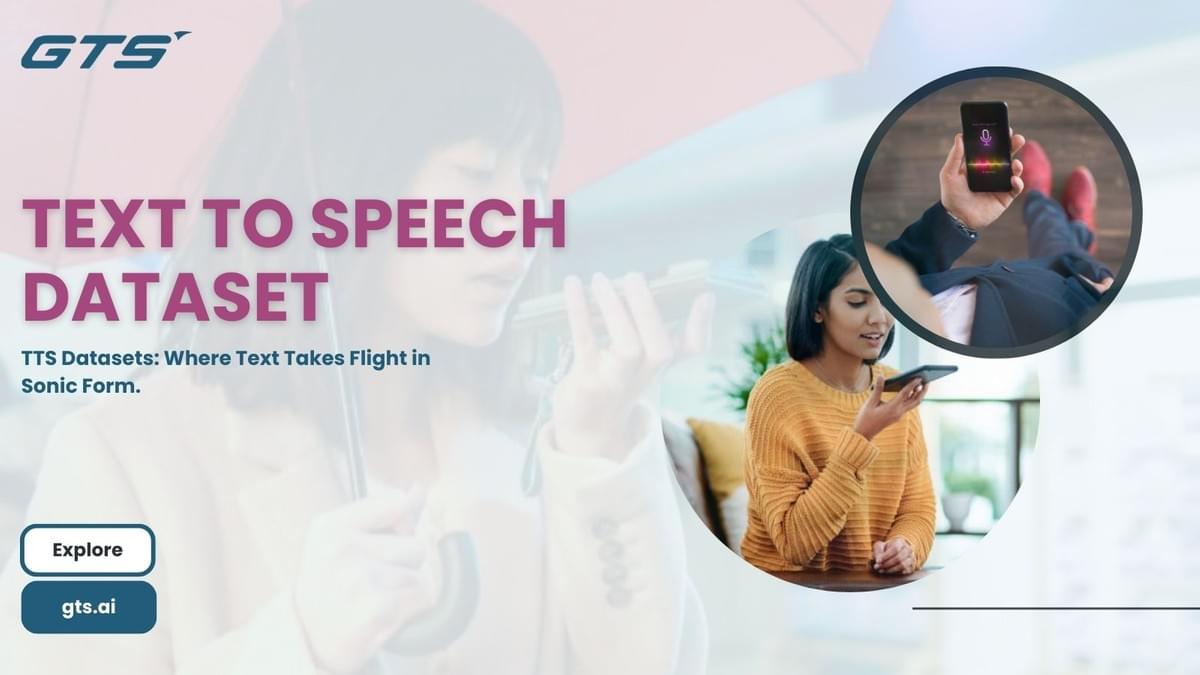
Introduction:
In the rapidly evolving landscape of artificial intelligence, the ability to convert text into natural-sounding human speech has become an indispensable technology. Text-To-Speech Dataset (TTS) systems have gained immense popularity due to their applications in accessibility, entertainment, virtual assistants, and more. At the heart of these systems lies the quality of the TTS dataset, which serves as the foundation for training models that can mimic the nuances of human speech. This blog, presented by Globose Technology Solutions Pvt Ltd (GTS), delves into the intricacies of designing effective text-to-speech training data, exploring the significance of high-quality datasets and their role in creating cutting-edge TTS systems.
The Crucial Role of TTS Datasets:
Creating a lifelike TTS system requires not only sophisticated machine learning algorithms but also a high-quality and diverse dataset. The dataset serves as a reference for the TTS model to learn the intricacies of human speech, including pronunciation, intonation, rhythm, and emotional nuances. A well-structured dataset is essential for ensuring that the TTS system produces speech that is not only intelligible but also natural-sounding and engaging to the listener.
Challenges in TTS Dataset Design:
Designing a comprehensive TTS dataset is not without its challenges. Some of the key challenges include:
- Variability: Human speech is highly variable, influenced by factors such as accents, dialects, speaking rates, and emotions. A diverse dataset that encompasses these variations is necessary to create a Text Data Collection system that can adapt to different contexts and user preferences.
- Data Collection: Curating a large and diverse dataset requires careful planning and data collection. This may involve collecting recordings from a range of speakers, covering different languages, ages, genders, and backgrounds.
- Annotation: Accurate phonetic and prosodic annotations are crucial for training TTS models effectively. Manual annotation is time-consuming and requires skilled linguists to mark stress patterns, intonation, and other speech nuances.
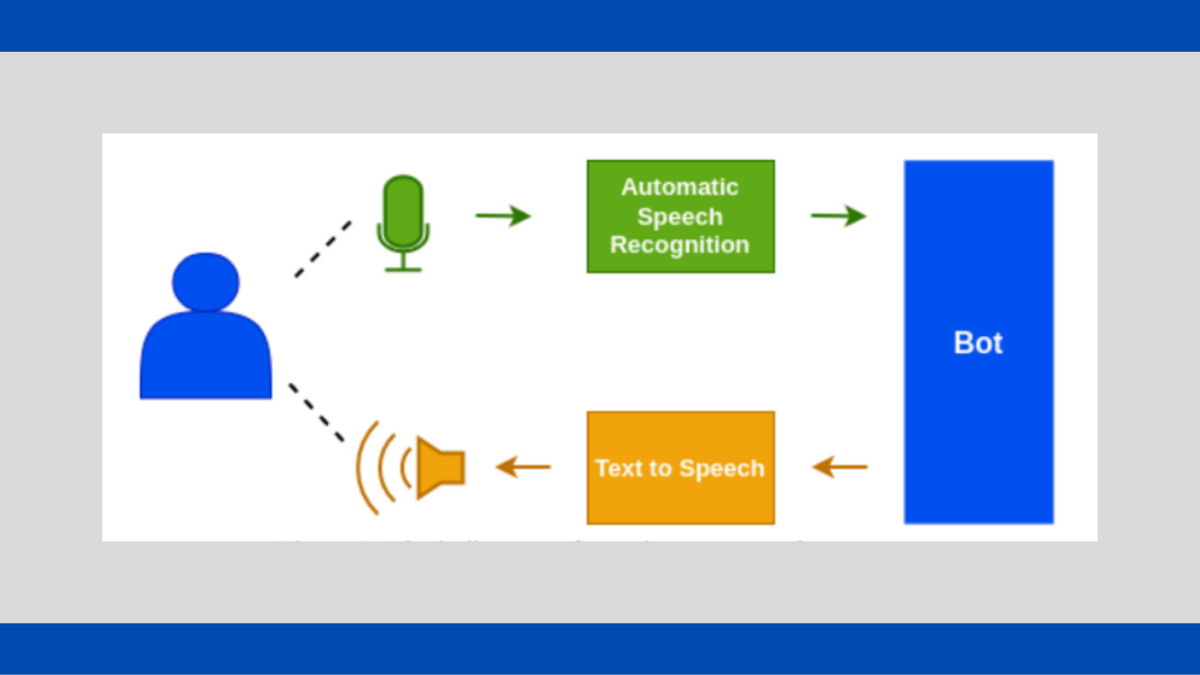
Creating High-Quality TTS Datasets:
Globose Technology Solutions Pvt Ltd (GTS) understands the significance of high-quality TTS datasets and employs a meticulous approach to dataset design:
- Speaker Diversity: GTS collects speech samples from a wide array of speakers to ensure that the TTS model can handle various vocal characteristics, from deep baritones to soft sopranos.
- Linguistic Coverage: The dataset includes a comprehensive range of phonemes and language styles to handle different languages, accents, and dialects.
- Emotional Variation: GTS captures emotional variation in speech, enabling the TTS model to infuse emotions into generated speech, making interactions more human-like.
- Naturalness: Recordings are made in natural speaking environments, capturing authentic speech patterns and rhythms.
The Impact on TTS System Performance:
The quality of the training dataset directly impacts the performance of the TTS system. A dataset with minimal biases, accurate annotations, and a wide variety of speech styles contributes to the creation of a TTS system that sets new benchmarks for naturalness, clarity, and expressiveness.
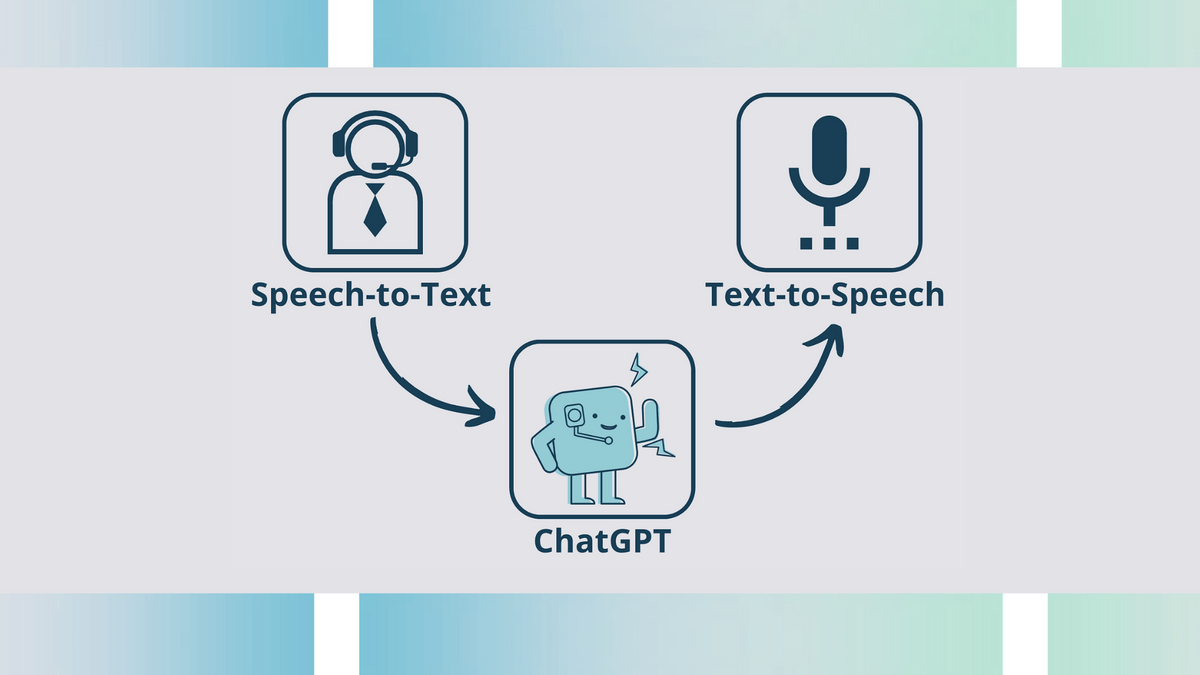
Conclusion:
Designing effective text-to-speech training data is an art that requires a deep understanding of human speech nuances, linguistic diversity, and technological advancements. Globose Technology Solutions Pvt Ltd (GTS) recognizes the pivotal role of high-quality datasets in shaping the next generation of TTS systems. By meticulously curating diverse and accurate datasets, GTS is at the forefront of developing TTS technology that not only mimics the human voice but also captures its essence, revolutionizing the way we interact with machines and technology