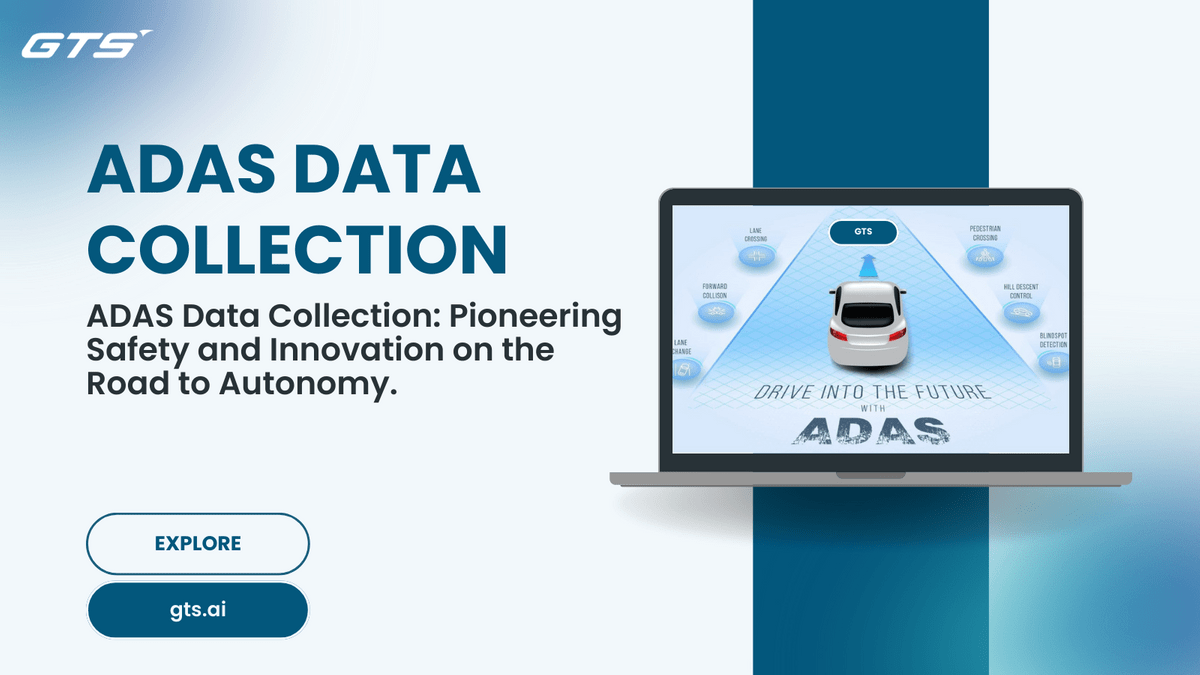
Introduction
Advanced Driver Assistance Systems (ADAS) have transformed the automotive industry by enhancing vehicle safety and comfort. These systems rely on a multitude of sensors, cameras, and radar systems to monitor the vehicle's surroundings, interpret data, and assist the driver in various ways. The heart and soul of these systems is data. The more data an ADAS Data Collection has access to, the smarter and more effective it becomes. In this blog, we'll explore the critical role data collection plays in developing and improving ADAS through machine learning.
The Growing Importance of ADAS
ADAS technologies encompass a broad range of features, including adaptive cruise control, lane-keeping assistance, blind-spot detection, and automated emergency braking. These systems have gained immense popularity for their ability to reduce accidents, make driving more convenient, and pave the way for fully autonomous vehicles. However, for ADAS to work effectively, they must collect and analyze vast amounts of data in real-time. This data enables them to make quick and precise decisions to ensure driver and passenger safety.
Data Sources for ADAS
To understand how ADAS work, it's essential to grasp the data sources that power them:
- Cameras: These capture images and videos of the vehicle's surroundings. They play a vital role in functions like lane detection, traffic sign recognition, and pedestrian detection.
- Lidar (Light Detection and Ranging): Lidar sensors emit laser pulses to measure distances between the sensor and surrounding objects. They create 3D maps of the environment and are instrumental in obstacle detection and mapping.
- Radar: Radar uses radio waves to detect the distance and speed of objects around the vehicle. It's particularly valuable for adaptive cruise control and collision avoidance.
- Ultrasonic Sensors: These sensors measure distances by emitting high-frequency sound waves and analyzing the reflected signals. They are often used for parking assistance and object detection at close range.
- GPS and Inertial Measurement Units (IMU): These provide information about the vehicle's location, speed, and direction, enabling functions like navigation, lane centering, and adaptive cruise control.
- Vehicle-to-Everything (V2X) Communication: This technology allows vehicles to communicate with each other and with infrastructure, sharing information about road conditions, traffic, and more.
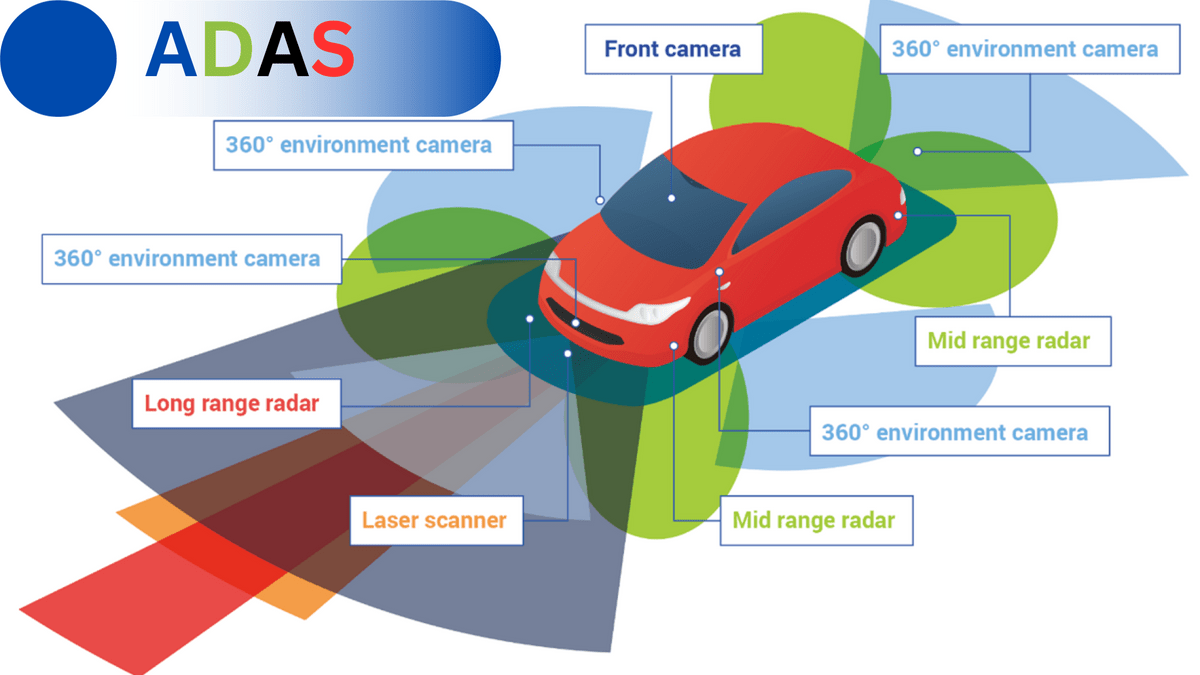
Data Collection and Machine Learning
Data collection is a continuous process in the development and improvement of ADAS. Machine learning, a subset of artificial intelligence, plays a significant role in making sense of this data. Let's delve deeper into how data collection and machine learning are intertwined in the context of ADAS Annotation:
- Data Labeling: To train machine learning algorithms, data must be labeled. This means that humans annotate the data to identify and classify objects, such as other vehicles, pedestrians, and road signs. Labeling is a labor-intensive process but is crucial for building accurate models.
- Training Data: Once data is labeled, it becomes training data for machine learning models. These models use this data to learn patterns and make predictions. The more diverse and extensive the training data, the better the models can perform.
- Real-Time Data Processing: ADAS systems operate in real-time, requiring the continuous processing of sensor data to make instantaneous decisions. Machine learning models must process and analyze this data swiftly and accurately.
- Predictive Algorithms: Machine learning algorithms predict events based on historical and real-time data. For example, an ADAS equipped with machine learning can predict when a collision is imminent and trigger emergency braking.
- Anomaly Detection: Machine learning models can identify anomalies in the data, which might indicate a malfunction or unsafe situation. For instance, sudden erratic behavior of a nearby vehicle could be flagged as an anomaly.
- Adaptive Behavior: ADAS systems equipped with machine learning can adapt to changing driving conditions. For instance, a vehicle's adaptive cruise control can adjust the following distance based on traffic flow and the driver's preferences.
Challenges in Data Collection for ADAS
Data collection for ADAS, while critical, is not without its challenges. Here are some of the key obstacles faced in this process:
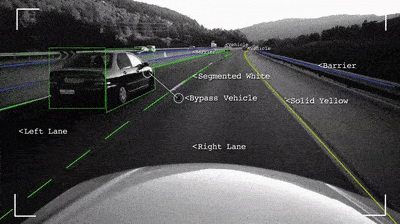
- Data Privacy and Security: Collecting data from vehicles raises concerns about privacy and data security. Striking the right balance between collecting valuable data and protecting driver privacy is essential.
- Data Quality: For machine learning to be effective, the quality of the data is paramount. Inaccurate or noisy data can lead to incorrect decisions and put safety at risk.
- Data Volume and Storage: ADAS systems generate enormous amounts of data, and storing and managing this data efficiently can be a logistical challenge.
- Labeling Labor: As mentioned earlier, data labeling is a labor-intensive task, requiring human annotators to mark objects in images and videos. Scaling this process can be time-consuming and costly.
- Adaptation to Varied Conditions: ADAS systems must adapt to diverse driving conditions, including different road types, weather, and traffic patterns. Ensuring the models are robust and reliable under all conditions is a complex task.
- Regulatory and Legal Considerations: Legal and regulatory frameworks for data collection and usage vary from one region to another. Manufacturers must navigate these complexities to deploy their systems globally.
Future Directions in ADAS Data Collection
As the automotive industry continues to evolve, so does the field of ADAS data collection. Here are some future directions and developments to look out for:
- Sensor Fusion: Combining data from multiple sensors, such as cameras, radar, and Lidar, will improve the accuracy and reliability of ADAS systems. Sensor fusion enables a more comprehensive understanding of the environment.
- 5G Connectivity: The rollout of 5G networks will enable faster and more reliable data transmission between vehicles and infrastructure, supporting real-time data exchange for V2X communication.
- Edge Computing: Moving some data processing closer to the vehicle (edge computing) can reduce latency and enhance the speed at which ADAS systems operate.
- Machine Learning Advances: As machine learning algorithms become more sophisticated, ADAS systems will be able to make even more nuanced decisions, improving safety and convenience.
- Regulatory Developments: Governments and regulatory bodies will continue to shape the way data is collected and used in the automotive industry. New regulations will influence data collection practices.
- Data Anonymization: Developing methods to collect valuable data without compromising driver privacy will be a priority. Anonymization techniques will help mitigate privacy concerns.
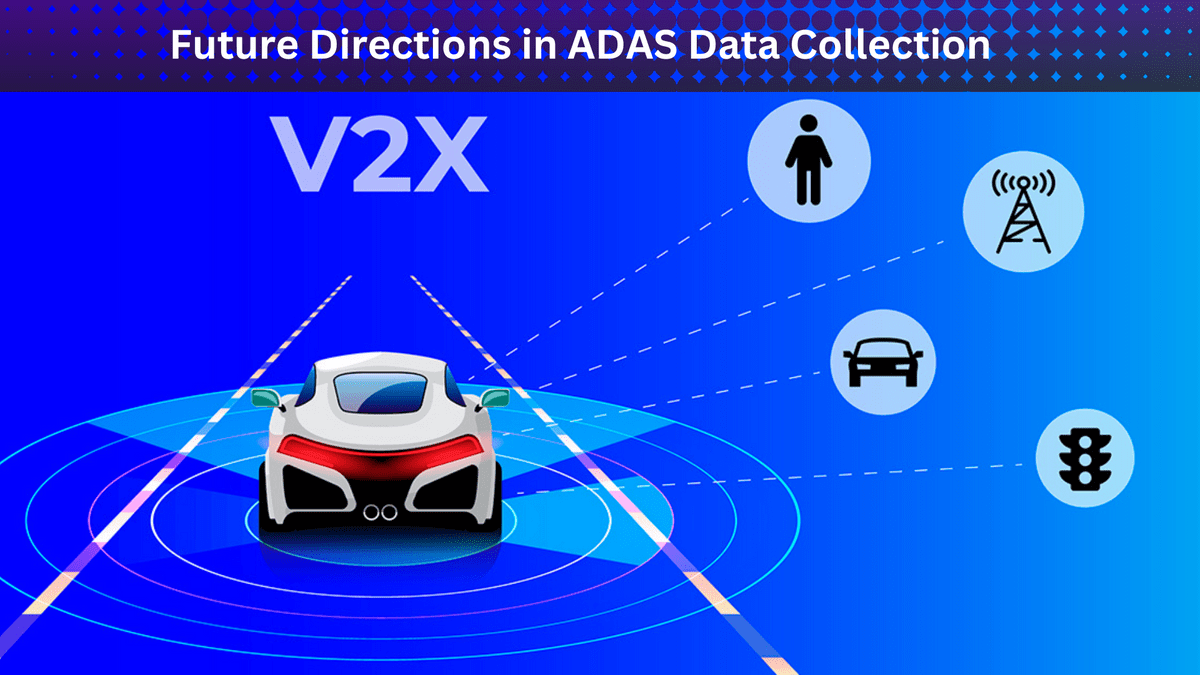
Conclusion
ADAS systems are at the forefront of vehicle safety and convenience, with data collection and machine learning as their foundation. These systems rely on a multitude of sensors and real-time data processing to make split-second decisions that enhance driving safety. Challenges such as data privacy, quality, and regulatory considerations must be addressed as the industry advances.
The future of ADAS is promising, with sensor fusion, 5G connectivity, edge computing, and advanced machine learning algorithms pushing the boundaries of what's possible. As these technologies continue to evolve, we can expect ADAS to become even more reliable, adaptable, and integral to the vehicles of tomorrow.
How GTS.AI Can Help You?
As vehicles evolve into intelligent entities, AI ADAS data collection emerges as a defining factor. Globose Technology Solutions Pvt Ltd (GTS) is at the forefront of this evolution, providing AI models with the data needed to make informed decisions, enhance safety, and revolutionize the driving experience. As we envision a future of autonomous mobility and connected vehicles, GTS's role in onboard intelligence through AI ADAS data collection remains an essential component of this transformative journey.