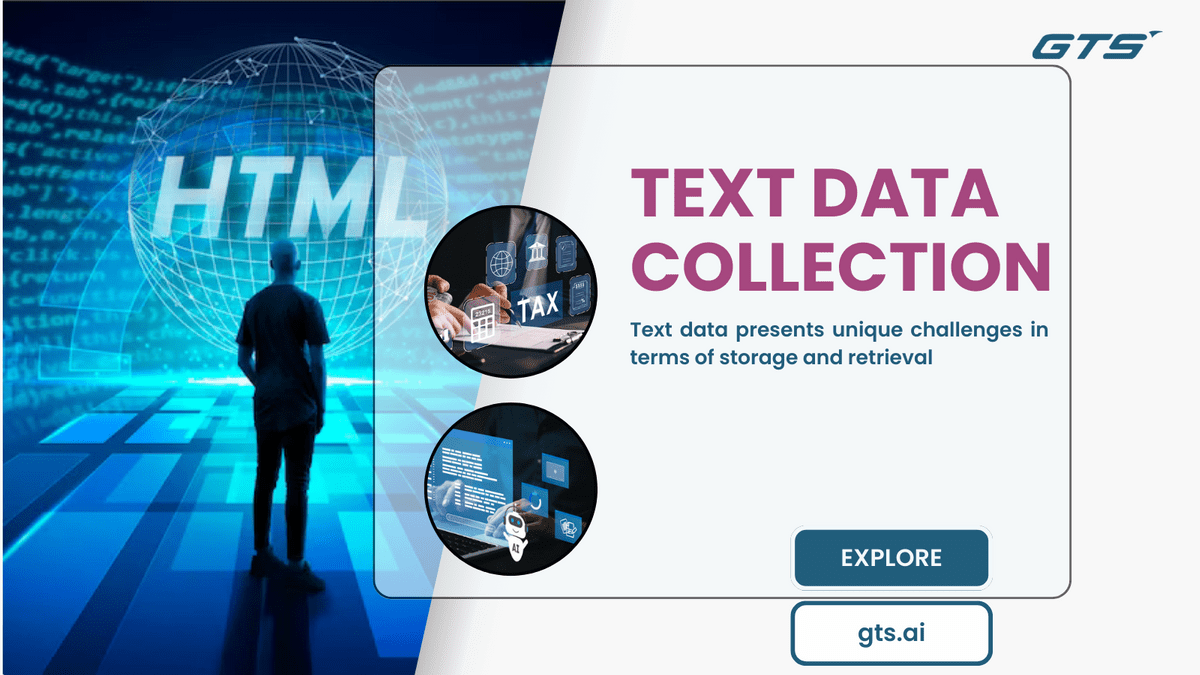
Introduction:
In the realm of artificial intelligence (AI) and machine learning (ML), the quality and relevance of data are paramount for building powerful and accurate models. When it comes to text data, efficient collection methods and careful curation are essential to unlock the full potential of AI algorithms. In this blog post, we will explore the winning formula for optimising AI Text Data Collection, enabling the creation of robust and high-performing ML models.
Understanding the Importance of Text Data Collection:
Textual information is ubiquitous in today's digital landscape. It encompasses a wide range of sources, such as social media posts, customer reviews, news articles, and scientific papers. Collecting and harnessing this data effectively is crucial for AI applications such as sentiment analysis, natural language processing, and text classification. The quality and diversity of the collected text data directly impact the accuracy and reliability of the resulting ML models.
Defining a Targeted Data Collection Strategy:
To optimise text data collection, it is essential to define a targeted strategy aligned with specific objectives. Here are some key considerations:
- Identifying Relevant Data Sources: Determine the sources of text data that are most relevant to your project's goals. These sources could include specific websites, social media platforms, or industry-specific databases.
- Data Filtering and Selection: Implement filters and criteria to ensure the collection of high-quality data. Define parameters such as language, date range, geographic location, or user demographics to narrow down the data to the most relevant and reliable sources.
- Sampling Techniques: Utilise sampling techniques to capture a representative subset of the overall text data. This approach helps in managing large volumes of data, reducing processing time, and optimising resource allocation.
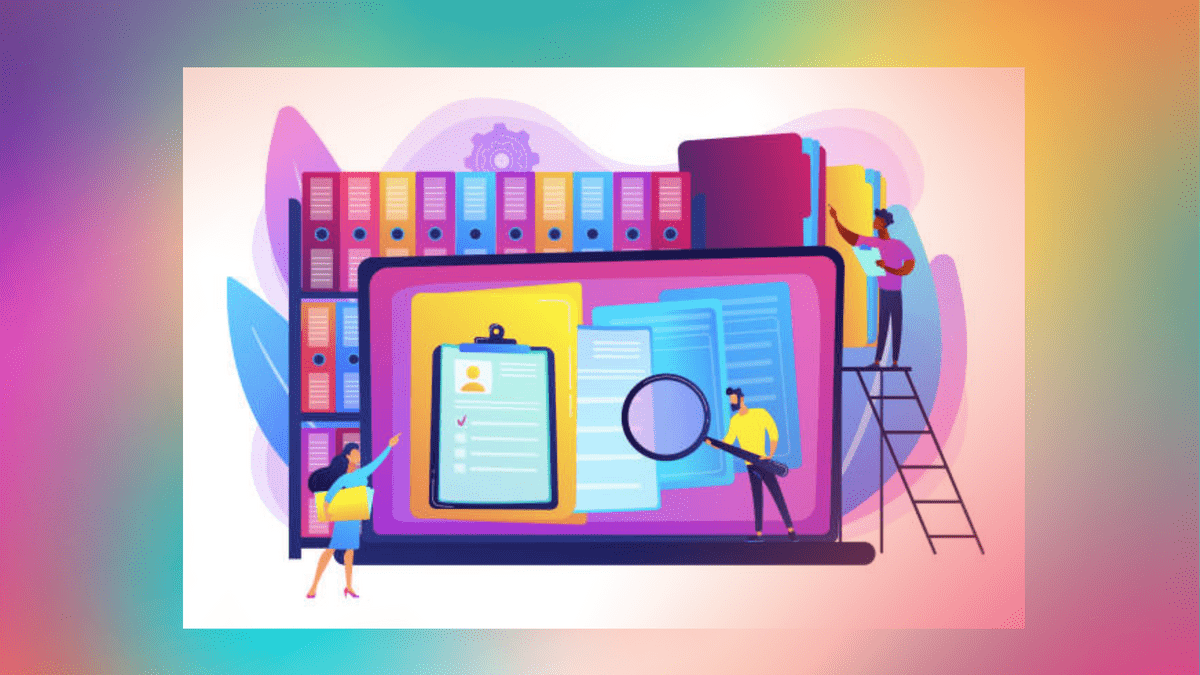
Leveraging Advanced Data Collection Tools and Techniques:
Maximising the efficiency and effectiveness of AI text data collection requires leveraging advanced tools and techniques. Here are some strategies to consider:
- Web Scraping: Web scraping tools can automate the extraction of text data from websites, saving time and effort. These tools allow for structured data collection, including text, Image Data Collection, metadata, and other relevant information.
- APIs and Data Feeds: Many online platforms and data providers offer APIs (Application Programming Interfaces) and data feeds that allow direct access to their text data. Leveraging these resources can simplify the data collection process and ensure up-to-date information.
- Natural Language Processing (NLP): NLP techniques, such as named entity recognition and topic modelling, can enhance the collection process by extracting specific information or identifying relevant topics from unstructured text data. These techniques help streamline data collection efforts and improve the quality of collected data.
Data Pre-processing and Annotation:
Optimising AI text data collection involves thorough pre-processing and annotation of the collected data. Key steps in this process include:
- Data Cleaning: Remove noise, irrelevant information, and inconsistencies from the collected text data. Techniques such as spell checking, removing stop words, and handling punctuation and special characters help ensure the data is clean and ready for analysis.
- Text Annotation: Add relevant labels, tags, or annotations to the collected text data to facilitate supervised learning. Annotation tasks could include sentiment labels, named entity recognition, or topic categorization. High-quality annotations are crucial for training accurate ML models.
Continuous Iteration and Improvement:
AI text data collection is an iterative process. It is important to continuously evaluate and refine the collection strategy based on the performance and feedback of ML models. By analysing the performance metrics and adjusting data collection techniques, organisations can improve the accuracy and efficacy of their models over time.
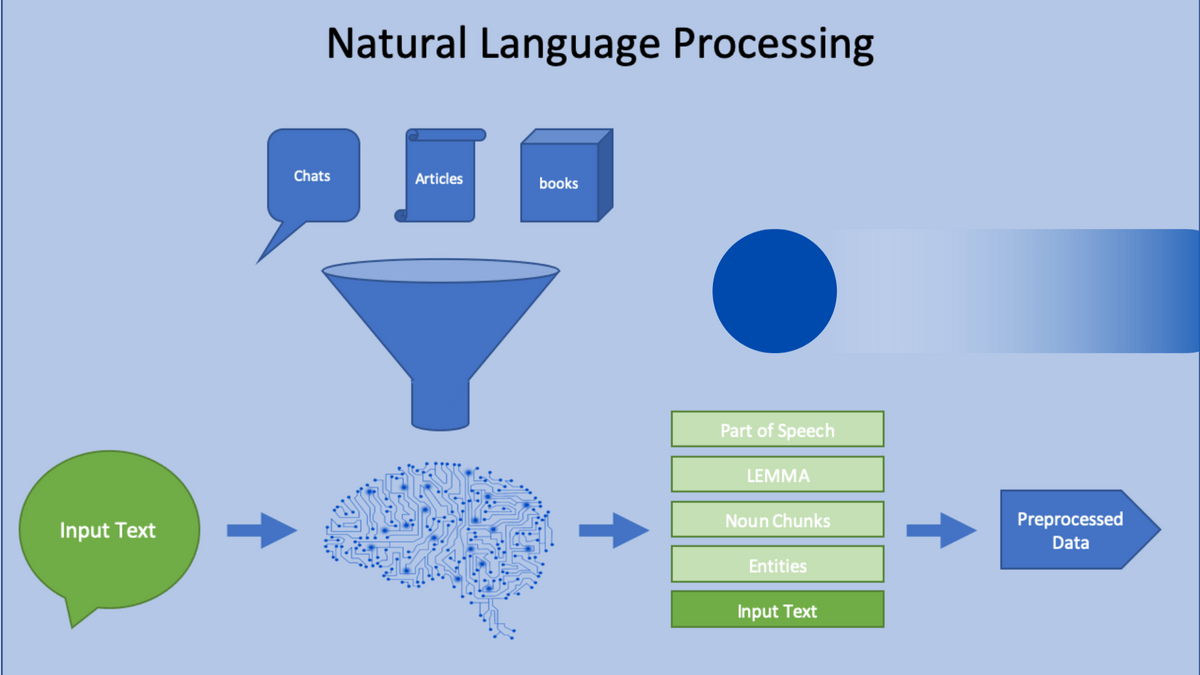
Conclusion:
Optimising AI text data collection is a winning formula for building powerful and accurate ML models. By defining a targeted strategy, leveraging advanced tools and techniques, and implementing thorough data pre-processing and annotation, organisations can unlock the true potential of text data. The synergy between well-curated text data and sophisticated ML algorithms creates a virtuous cycle, where the models improve with each iteration, leading to better insights and more informed decision-making.
How GTS.AI can be a right Text Data Collection
Globose Technology Solutions can be a right text data collection because it contains a vast and diverse range of text data that can be used for various naturals language processing tasks,including machine learning ,text classification,sentiment analysis,topic modeling ,Image Data Collection and many others. It provides a large amount of text data in multiple languages,including English,spanish,french,german,italian,portuguese,dutch, russian,chinese,and many others.In conclusion, the importance of quality data in text collection for machine learning cannot be overstated. It is essential for building accurate, reliable, and robust natural language processing models.